Business
This week in AI: AI ethics keeps falling by the wayside
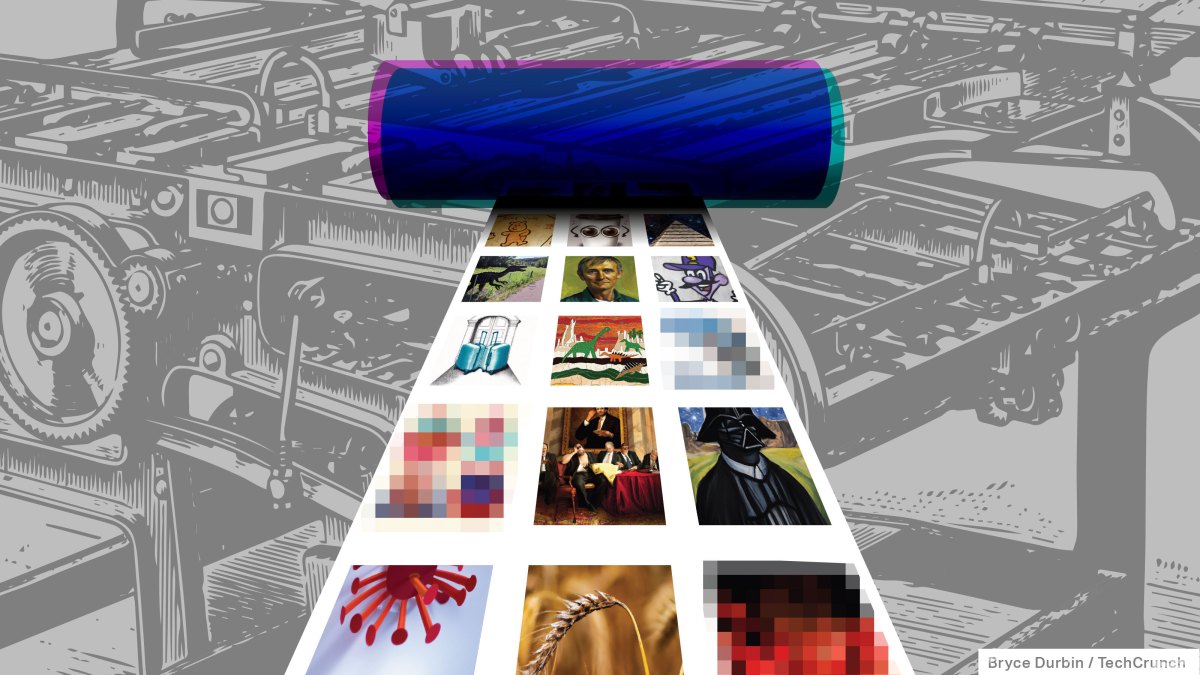
Keeping up with an industry as fast-moving as AI is a tall order. So until an AI can do it for you, here’s a handy roundup of recent stories in the world of machine learning, along with notable research and experiments we didn’t cover on their own.
This week in AI, the news cycle finally (finally!) quieted down a bit ahead of the holiday season. But that’s not to suggest there was a dearth to write about, a blessing and a curse for this sleep-deprived reporter.
A particular headline from the AP caught my eye this morning: “AI image-generators are being trained on explicit photos of children.” The gist of the story is, LAION, a data set used to train many popular open source and commercial AI image generators, including Stable Diffusion and Imagen, contains thousands of images of suspected child sexual abuse. A watchdog group based at Stanford, the Stanford Internet Observatory, worked with anti-abuse charities to identify the illegal material and report the links to law enforcement.
Now, LAION, a nonprofit, has taken down its training data and pledged to remove the offending materials before republishing it. But incident serves to underline just how little thought is being put into generative AI products as the competitive pressures ramp up.
Thanks to the proliferation of no-code AI model creation tools, it’s becoming frightfully easy to train generative AI on any data set imaginable. That’s a boon for startups and tech giants alike to get such models out the door. With the lower barrier to entry, however, comes the temptation to cast aside ethics in favor of an accelerated path to market.
Ethics is hard — there’s no denying that. Combing through the thousands of problematic images in LAION, to take this week’s example, won’t happen overnight. And ideally, developing AI ethically involves working with all relevant stakeholders, including organizations who represent groups often marginalized and adversely impacted by AI systems.
The industry is full of examples of AI release decisions made with shareholders, not ethicists, in mind. Take for instance Bing Chat (now Microsoft Copilot), Microsoft’s AI-powered chatbot on Bing, which at launch compared a journalist to Hitler and insulted their appearance. As of October, ChatGPT and Bard, Google’s ChatGPT competitor, were still giving outdated, racist medical advice. And the latest version of OpenAI’s image generator DALL-E shows evidence of Anglocentrism.
Suffice it to say harms are being done in the pursuit of AI superiority — or at least Wall Street’s notion of AI superiority. Perhaps with the passage of the EU’s AI regulations, which threaten fines for noncompliance with certain AI guardrails, there’s some hope on the horizon. But the road ahead is long indeed.
Here are some other AI stories of note from the past few days:
Predictions for AI in 2024: Devin lays out his predictions for AI in 2024, touching on how AI might impact the U.S. primary elections and what’s next for OpenAI, among other topics.
Against pseudanthropy: Devin also wrote suggesting that AI be prohibited from imitating human behavior.
Microsoft Copilot gets music creation: Copilot, Microsoft’s AI-powered chatbot, can now compose songs thanks to an integration with GenAI music app Suno.
Facial recognition out at Rite Aid: Rite Aid has been banned from using facial recognition tech for five years after the Federal Trade Commission found that the U.S. drugstore giant’s “reckless use of facial surveillance systems” left customers humiliated and put their “sensitive information at risk.”
EU offers compute resources: The EU is expanding its plan, originally announced back in September and kicked off last month, to support homegrown AI startups by providing them with access to processing power for model training on the bloc’s supercomputers.
OpenAI gives board new powers: OpenAI is expanding its internal safety processes to fend off the threat of harmful AI. A new “safety advisory group” will sit above the technical teams and make recommendations to leadership, and the board has been granted veto power.
Q&A with UC Berkeley’s Ken Goldberg: For his regular Actuator newsletter, Brian sat down with Ken Goldberg, a professor at UC Berkeley, a startup founder and an accomplished roboticist, to talk humanoid robots and broader trends in the robotics industry.
CIOs take it slow with gen AI: Ron writes that, while CIOs are under pressure to deliver the kind of experiences people are seeing when they play with ChatGPT online, most are taking a deliberate, cautious approach to adopting the tech for the enterprise.
News publishers sue Google over AI: A class action lawsuit filed by several news publishers accuses Google of “siphon[ing] off” news content through anticompetitive means, partly through AI tech like Google’s Search Generative Experience (SGE) and Bard chatbot.
OpenAI inks deal with Axel Springer: Speaking of publishers, OpenAI inked a deal with Axel Springer, the Berlin-based owner of publications including Business Insider and Politico, to train its generative AI models on the publisher’s content and add recent Axel Springer-published articles to ChatGPT.
Google brings Gemini to more places: Google integrated its Gemini models with more of its products and services, including its Vertex AI managed AI dev platform and AI Studio, the company’s tool for authoring AI-based chatbots and other experiences along those lines.
More machine learnings
Certainly the wildest (and easiest to misinterpret) research of the last week or two has to be life2vec, a Danish study that uses countless data points in a person’s life to predict what a person is like and when they’ll die. Roughly!

Visualization of the life2vec’s mapping of various relevant life concepts and events.
The study isn’t claiming oracular accuracy (say that three times fast, by the way) but rather intends to show that if our lives are the sum of our experiences, those paths can be extrapolated somewhat using current machine learning techniques. Between upbringing, education, work, health, hobbies, and other metrics, one may reasonably predict not just whether someone is, say, introverted or extroverted, but how these factors may affect life expectancy. We’re not quite at “precrime” levels here but you can bet insurance businesses can’t wait to license this work.
Another big claim was made by CMU scientists who created a system called Coscientist, an LLM-based assistant for researchers that can do a lot of lab drudgery autonomously. It’s limited to certain domains of chemistry currently, but just like scientists, models like these will be specialists.
Lead researcher Gabe Gomes told Nature: “The moment I saw a non-organic intelligence be able to autonomously plan, design and execute a chemical reaction that was invented by humans, that was fantastic. It was a ‘holy crap’ moment.” Basically it uses an LLM like GPT-4, fine tuned on chemistry documents, to identify common reactions, reagents, and procedures and perform them. So you don’t need to tell a lab tech to synthesize 4 batches of some catalyst — the AI can do it, and you don’t even need to hold its hand.
Google’s AI researchers have had a big week as well, diving into a few interesting frontier domains. FunSearch may sound like Google for kids, but it actually is short for function search, which like Coscientist is able to make and help make mathematical discoveries. Interestingly, to prevent hallucinations, this (like others recently) use a matched pair of AI models a lot like the “old” GAN architecture. One theorizes, the other evaluates.
While FunSearch isn’t going to make any ground-breaking new discoveries, it can take what’s out there and hone or reapply it in new places, so a function that one domain uses but another is unaware of might be used to improve an industry standard algorithm.
StyleDrop is a handy tool for people looking to replicate certain styles via generative imagery. The trouble (as the researcher see it) is that if you have a style in mind (say “pastels”) and describe it, the model will have too many sub-styles of “pastels” to pull from, so the results will be unpredictable. StyleDrop lets you provide an example of the style you’re thinking of, and the model will base its work on that — it’s basically super-efficient fine-tuning.

Image Credits: Google
The blog post and paper show that it’s pretty robust, applying a style from any image, whether it’s a photo, painting, cityscape or cat portrait, to any other type of image, even the alphabet (notoriously hard for some reason).
Google is also moving along in the generative video game with VideoPoet, which uses an LLM base (like everything else these days… what else are you going to use?) to do a bunch of video tasks, turning text or images to video, extending or stylizing existing video, and so on. The challenge here, as every project makes clear, is not simply making a series of images that relate to one another, but making them coherent over longer periods (like more than a second) and with large movements and changes.
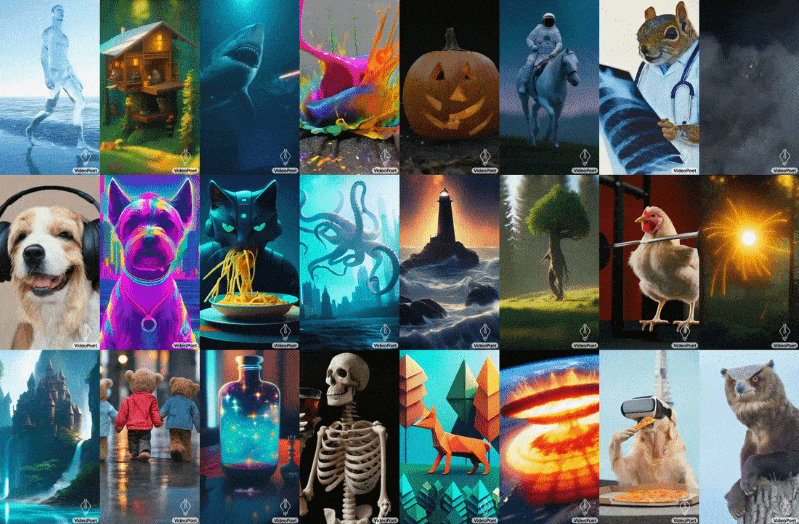
Image Credits: Google
VideoPoet moves the ball forward, it seems, though as you can see the results are still pretty weird. But that’s how these things progress: first they’re inadequate, then they’re weird, then they’re uncanny. Presumably they leave uncanny at some point but no one has really gotten there yet.
On the practical side of things, Swiss researchers have been applying AI models to snow measurement. Normally one would rely on weather stations, but these can be far between and we have all this lovely satellite data, right? Right. So the ETHZ team took public satellite imagery from the Sentinel-2 constellation, but as lead Konrad Schindler puts it, “Just looking at the white bits on the satellite images doesn’t immediately tell us how deep the snow is.”
So they put in terrain data for the whole country from their Federal Office of Topography (like our USGS) and trained up the system to estimate not just based on white bits in imagery but also ground truth data and tendencies like melt patterns. The resulting tech is being commercialized by ExoLabs, which I’m about to contact to learn more.
A word of caution from Stanford, though — as powerful as applications like the above are, note that none of them involve much in the way of human bias. When it comes to health, that suddenly becomes a big problem, and health is where a ton of AI tools are being tested out. Stanford researchers showed that AI models propagate “old medical racial tropes.” GPT-4 doesn’t know whether something is true or not, so it can and does parrot old, disproved claims about groups, such as that black people have lower lung capacity. Nope! Stay on your toes if you’re working with any kind of AI model in health and medicine.
Lastly, here’s a short story written by Bard with a shooting script and prompts, rendered by VideoPoet. Watch out, Pixar!
-
Entertainment7 days ago
I went to the ‘Severance’ pop-up in Grand Central Station. It was wild.
-
Entertainment6 days ago
What’s new to streaming this week? (Jan. 17, 2025)
-
Entertainment6 days ago
Explainer: Age-verification bills for porn and social media
-
Entertainment5 days ago
If TikTok is banned in the U.S., this is what it will look like for everyone else
-
Entertainment5 days ago
‘Night Call’ review: A bad day on the job makes for a superb action movie
-
Entertainment5 days ago
How ‘Grand Theft Hamlet’ evolved from lockdown escape to Shakespearean success
-
Entertainment5 days ago
‘September 5’ review: a blinkered, noncommittal thriller about an Olympic hostage crisis
-
Entertainment5 days ago
‘Back in Action’ review: Cameron Diaz and Jamie Foxx team up for Gen X action-comedy